Table Of Content
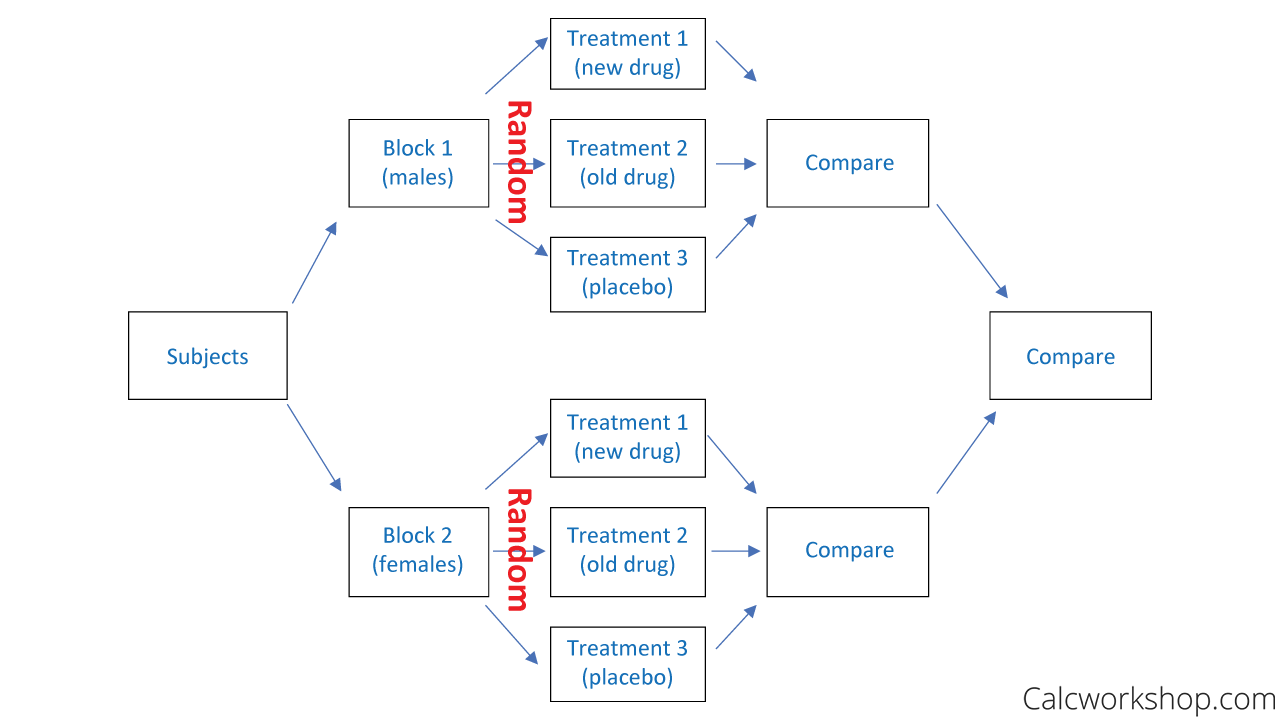
While such designs are commonly and successfully used, especially in smaller experiments, their application can often be unrealistic or impractical in many settings. Let’s start with the basic 22 factorial design to introduce the effective use of blocking into the 2k design (Table 1). Let’s assume that we need at least three replications for this particular experiment. If one batch can produce enough raw materials for only four samples (experimental units), only one replication can be made from one batch. Therefore, three batches will be required to complete the three full replications for the 22 basic factorial design (Table 2).
Mitigation of Batch Effects
If we choose one of the RCB Design Structures, Temperature effects are completely randomized at the Run level. Recipe is nested within temperature and has a different error structure than temperature, because each dough appears within each run. The contrasts looking at recipe and recipe by dough non-additivity (interaction) do not have run-to-run variability in them.
Batch
More traffic experiments planned in Fayetteville - Fayetteville Flyer
More traffic experiments planned in Fayetteville.
Posted: Fri, 21 Jul 2017 07:00:00 GMT [source]
Such interaction is likely caused by other biologically relevant factors that influence the effect of (some) treatments but that have not been accounted for in our experiment and analysis. It is important to distribute, as best one can,the different levelsof the treatment variables equally over the different levels of thecontrol variables. In case of substantial confounding, as when mostof the subjects receiving Placebo are Female and most of those receiving the Treatment are Male, it can become impossible to estimate the treatmenteffect. Similarly, when all Treatment subjects arein one batch and all Placebo in the other, batchand treatment are confounded and without a batch-independent referenceit is not possible to distinguish the treatment effect from a possiblebatch effect. Thus, it is important to account for control variablesin the experimental design and in the sample organization, and thiscan also be achieved using block randomization. The only systematic (non-random) differences in the observed responses result from differences between the treatments.
Missing Data

The blocking and confounding techniques in 2k factorial design of experiment is described in Video 1. In that sense, Latin Square designs are useful building blocksof more complex designs, see for example Kuehl (2000). In the most basic form, we assume that we do not have replicateswithin a block.
As we shall see, Latin squares can be used as much as the RCBD in industrial experimentation as well as other experiments. When we have a single blocking factor available for our experiment we will try to utilize a randomized complete block design (RCBD). We also consider extensions when more than a single blocking factor exists which takes us to Latin Squares and their generalizations. When we can utilize these ideal designs, which have nice simple structure, the analysis is still very simple, and the designs are quite efficient in terms of power and reducing the error variation. The single design we looked at so far is the completely randomized design (CRD) where we only have a single factor. In the CRD setting we simply randomly assign the treatments to the available experimental units in our experiment.
Robust parameter design is widely used as an effective tool to reduce process variability by appropriate selection of control factors to make the process insensitive to noise. In this paper, we propose and study a method for selecting the optimal blocked robust parameter designs when some of the control-by-noise interactions are included in the model. We then discuss how to search for the best designs according to this method and present some results for designs of 8 and 16 runs.
The row effect is the order of treatment, whether A is done first or second or whether B is done first or second. So, if we have 10 subjects we could label all 10 of the subjects as we have above, or we could label the subjects 1 and 2 nested in a square. This is similar to the situation where we have replicated Latin squares - in this case five reps of 2 × 2 Latin squares, just as was shown previously in Case 2. Consider a factory setting where you are producing a product with 4 operators and 4 machines.
2.3 Contrasts
A block is characterized by a set of homogeneous plots or a set of similar experimental units. In agriculture a typical block is a set of contiguous plots of land under the assumption that fertility, moisture, weather, will all be similar, and thus the plots are homogeneous. The first step of implementing blocking is deciding what variables you need to balance across your treatment groups. We should keep in mind that variance components are harder to estimate than averages, and estimates based on low replication are imprecise. This is not a problem if our goal is removal of variation from treatment contrasts, but sufficient replication is necessary if estimation of variance components is a primary goal of the experiment. Sample size determination for variance component estimation is covered in more specialized texts.
Blackboxes (left) indicate Placebo, red boxes (right)indicate Treatment. The “true abundance”is what would have been measured without the machine drift (Figure Figure11, second row). The“ordered allocation”, “complete randomization”,and “block randomization” is as depicted in Figure Figure11D, H, and L, respectively. Interpretation of the coefficients of the corresponding models, residualanalysis, etc. is done “as usual.” The only difference is that we do not test theblock factor for statistical significance, but for efficiency.
In practice, it is notalways possible or preferable to have thesame number of subjects in all groups of the treatment variable(s).18,19 The block randomization procedure with groups of different sizesis only slightly different. As before, first, blocks of samples whereeach group is proportionally represented are created. For example,if one group is twice as large as the other group, each block wouldconsist of three subjects, one of the smaller group and two of thelarger group (Figure Figure33A).
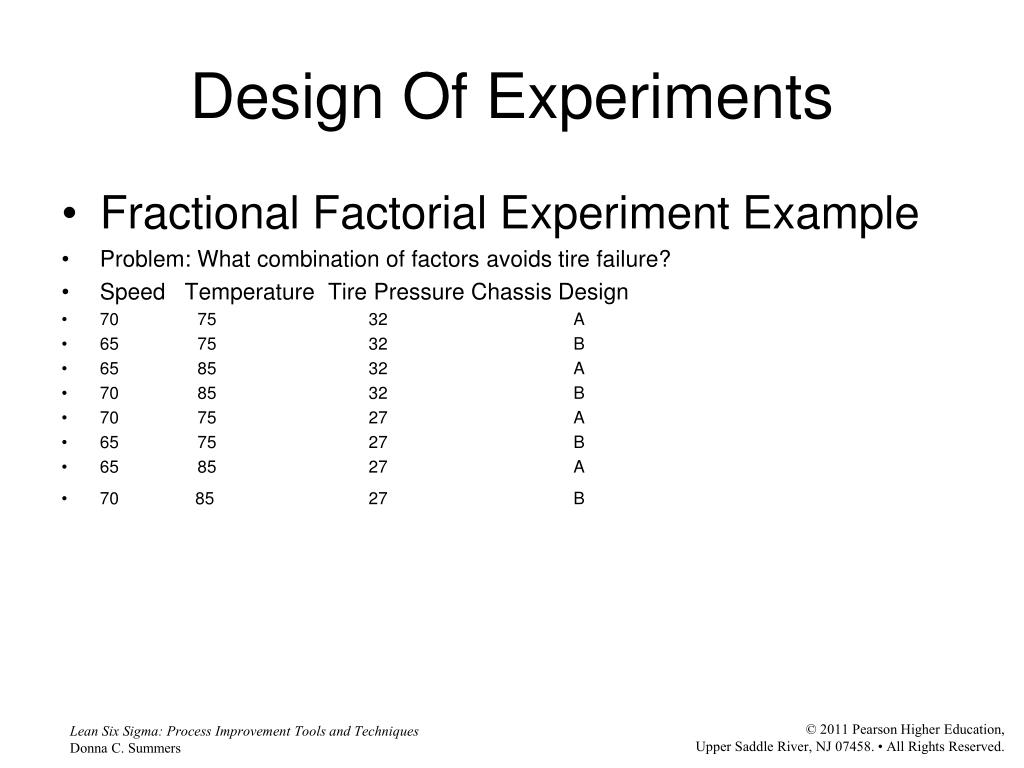
A common choice for blocking mice is by litter, since sibling mice often show more similar responses as compared to mice from different litters (Perrin 2014). Litter sizes are typically in the range of 5–7 animals in mice (Watt 1934), which would easily allow us to select three mice from each litter for our experiment. In contrast to a randomized complete block design, we cannot increase the number of blocks to increase the replication. With two levels of Sex fixed, we instead need to increase the experiment size by using multiple mice of each sex for each drug. Since Sex and Drug are fixed, so is their interaction, and all fixed factors are tested against the same error term from (Mouse). Each treatment occurs only once per block, and the variations due to interactions and residuals are completely confounded.
The degrees of freedom for error grows very rapidly when you replicate Latin squares. But usually if you are using a Latin Square then you are probably not worried too much about this error. The error is more dependent on the specific conditions that exist for performing the experiment. For instance, if the protocol is complicated and training the operators so they can conduct all four becomes an issue of resources then this might be a reason why you would bring these operators to three different factories. It depends on the conditions under which the experiment is going to be conducted.
A similar exercise can be done to illustrate the confounded situation where the main effect, say A, is confounded with blocks. Again, since this is a bit nonstandard, we will need to generate a design in Minitab using the default settings and then edit the worksheet to create the confounding we desire and analyze it in GLM. When I have time to think it through, I'll update this further with the appropriate fancy names for those experiment designs. You are studying how bread dough and baking temperature affect the tastiness of bread.
A special case is the so-calledLatin Square design where we have two blockfactors and one treatment factor having \(g\) levels each (yes, all of them!).Hence, this is a very restrictive assumption. In a Latin Square design, eachtreatment (Latin letters) appears exactly once in each row and once ineach column. If we ignore the columns of a Latin Square designs, the rows form anRCBD; if we ignore the rows, the columns form an RCBD.
No comments:
Post a Comment